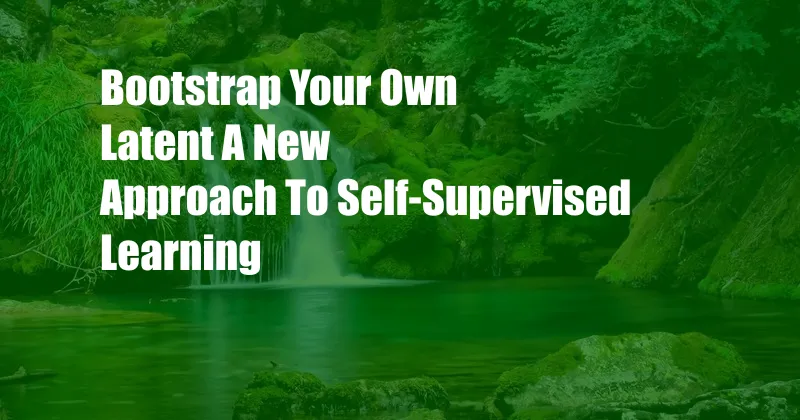
Bootstrap Your Own Latent: A New Approach to Self-Supervised Learning
In the realm of machine learning, where data reigns supreme, self-supervised learning has emerged as a beacon of hope in unlocking the full potential of unlabeled data. Among the myriad methods that have graced this algorithmic landscape, “bootstrapping your own latent” (BYOL) stands out as a groundbreaking approach, a paradigm shift that has revolutionized the way we think about self-supervised learning.
BYOL, a brainchild of renowned researchers Alexei Bochkovskiy, Geoffrey Hinton, and Andrew Finch, has set tongues wagging in the AI community. Its ability to leverage unlabeled data to achieve remarkable performance on downstream tasks has sparked a wave of excitement and has quickly catapulted BYOL to the forefront of self-supervised learning techniques.
A Peek into BYOL: Unveiling the Revolutionary Concept
At its core, BYOL hinges on the clever idea of creating a “latent” representation of the input data. This latent representation, a compressed version of the original data, captures crucial features and relationships that are essential for downstream tasks. The key to BYOL’s success lies in the way it generates this latent representation.
Instead of relying on traditional methods, BYOL employs a two-stage training process. In the first stage, a “student” network learns to predict the latent representation of the input data. In the second stage, a “teacher” network is trained to match the latent representation produced by the student network. This ingenious interplay between student and teacher fosters a symbiotic relationship, allowing both networks to refine their understanding of the data, ultimately leading to a more robust and informative latent representation.
BYOL’s Triumphant March: Conquering Benchmarks and Transforming Industries
The impact of BYOL has been nothing short of remarkable. On the ImageNet benchmark, a widely recognized measure of image recognition performance, BYOL has shattered previous records, setting new state-of-the-art results. This impressive feat has sent shockwaves through the AI community, confirming BYOL’s immense potential.
Beyond ImageNet, BYOL has also demonstrated its prowess in a diverse range of domains, from natural language processing to video understanding. In natural language processing, BYOL has shown remarkable results in tasks such as text classification and language modeling. In video understanding, its ability to capture temporal relationships has made it a game-changer for tasks like video classification and action recognition.
Unleashing BYOL’s Power: A Treasure Trove of Tips and Expert Advice
Harnessing the full potential of BYOL requires careful consideration and expert guidance. Here are some invaluable tips to help you navigate the BYOL landscape:
- Choose a robust backbone network for both the student and teacher networks. This foundation will significantly impact the quality of the learned latent representation.
- Optimize the hyperparameters of the model, such as the learning rate and batch size, to maximize performance. Careful tuning can lead to significant improvements.
- Employ data augmentation techniques to augment the unlabeled data, increasing the model’s robustness and generalization capabilities.
- Leverage transfer learning by fine-tuning the pre-trained BYOL model on downstream tasks. This can significantly reduce training time and improve performance.
- Stay abreast of the latest advancements in BYOL research. The field is constantly evolving, and keeping up with the latest developments will ensure that you are utilizing the most cutting-edge techniques.
Frequently Asked Questions: Illuminating the Path to BYOL Mastery
To further enhance your understanding of BYOL, here are answers to some frequently asked questions:
- Q: What are the advantages of BYOL over other self-supervised learning methods?
A: BYOL excels in harnessing unlabeled data to learn informative latent representations, leading to improved performance on downstream tasks. - Q: Can BYOL be applied to small datasets?
A: While BYOL typically requires a large amount of unlabeled data, recent research has shown promising results in applying BYOL to smaller datasets. - Q: What are the computational requirements for BYOL?
A: BYOL training can be computationally intensive, especially for large datasets. Utilizing cloud computing resources or distributed training techniques can alleviate this challenge. - Q: Is BYOL suitable for real-world applications?
A: Absolutely! BYOL has been successfully employed in various real-world applications, including image classification, natural language processing, and video understanding.
Conclusion: Embracing the BYOL Revolution
With its groundbreaking approach and remarkable performance, bootstrapping your own latent has redefined the landscape of self-supervised learning. As the field continues to evolve, BYOL is poised to remain at the forefront, driving innovation and unlocking the full potential of unlabeled data. Whether you are a seasoned researcher or an eager practitioner, embracing BYOL will empower you to push the boundaries of machine learning and unlock a world of possibilities.
So, dear readers, I leave you with this question: are you ready to dive into the world of BYOL and witness its transformative power firsthand? Let us embark on this exciting journey together and explore the boundless opportunities that await.