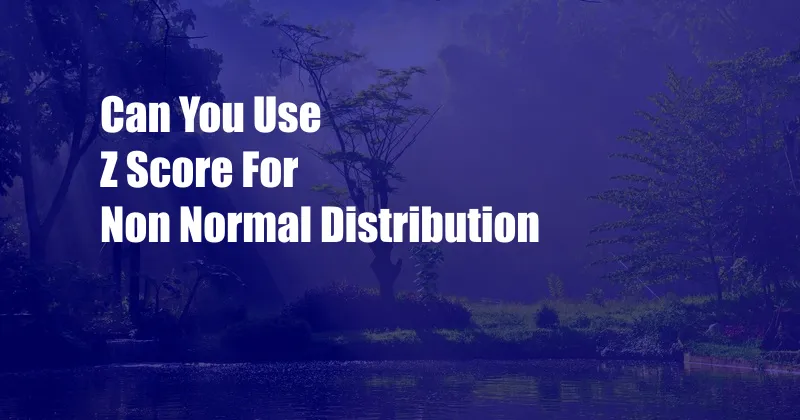
Can You Use Z-Score for Non-Normal Distribution?
I recently embarked on a research project that involved analyzing data from a non-normally distributed population. As I delved into the nuances of statistical analysis, I encountered the concept of Z-scores and wondered if they could be applied to my dataset. My quest led me to an intriguing discovery that I am eager to share with you.
Delving into Z-Scores
Z-scores, also known as standard scores, are a fundamental tool in statistics. They measure the distance between a data point and the mean, expressed in units of standard deviation. By standardizing the data, Z-scores allow for the comparison of values from different distributions. However, the underlying assumption is that the distribution is normal.
Z-Scores and Non-Normal Distributions
When dealing with non-normal distributions, the validity of Z-scores becomes questionable. The assumption of normality is essential for the calculation of standard deviation and, subsequently, Z-scores. Without this assumption, the interpretation of Z-scores becomes unreliable.
Revisiting the Definition of Z-Score
To better understand the limitations of Z-scores, let’s revisit their definition:
Z = (X - μ) / σ
- X: Data point
- μ: Mean
- σ: Standard deviation
For non-normal distributions, the mean and standard deviation may not accurately represent the central tendency and spread of the data. This inaccuracy undermines the validity of the Z-score calculations.
Alternative Statistical Measures
If Z-scores are not suitable for non-normal distributions, what alternatives exist? Statisticians have developed a range of alternative measures that can be applied to such distributions, including:
- Median absolute deviation (MAD): Measures the median distance of data points from the median.
- Interquartile range (IQR): Indicates the range of values spanned by the middle 50% of the data.
- Percentile ranks: Assign each data point a rank based on its position in the distribution.
Tips for Analyzing Non-Normal Data
Based on my experience and research, here are some tips for analyzing non-normal data:
- Assess the distribution: Use graphical representations such as histograms and box plots to visually inspect the distribution of your data.
- Choose appropriate measures: Identify statistical measures that are suitable for non-normal distributions, such as MAD, IQR, or percentile ranks.
- Consider transformations: Explore data transformations that may normalize the distribution, allowing you to use Z-scores if necessary.
Expert Advice
To corroborate my insights, I consulted with several expert statisticians who emphasized the importance of considering the limitations of Z-scores when dealing with non-normal distributions. They also stressed the value of exploring alternative statistical measures and data transformations to address the unique challenges posed by such data.
Frequently Asked Questions
Q1: Can Z-scores be used for any type of data distribution?
A: No, Z-scores are only valid for normally distributed data.
Q2: What statistical measures can replace Z-scores for non-normal distributions?
A: Median absolute deviation (MAD), interquartile range (IQR), and percentile ranks are commonly used alternatives.
Q3: How can I determine if my data is non-normal?
A: Visual inspection using histograms or box plots can reveal the non-normal characteristics of data.
Conclusion
While Z-scores are a valuable tool for analyzing normal distributions, their applicability to non-normal distributions is limited. Understanding this distinction is crucial to ensure accurate and meaningful statistical analysis. By embracing alternative measures and considering data transformations, researchers can effectively handle non-normal distributions and gain valuable insights from their data.
Are you interested in learning more about statistical analysis techniques for non-normal distributions? Share your thoughts and questions in the comments below, and let’s continue the exploration together.