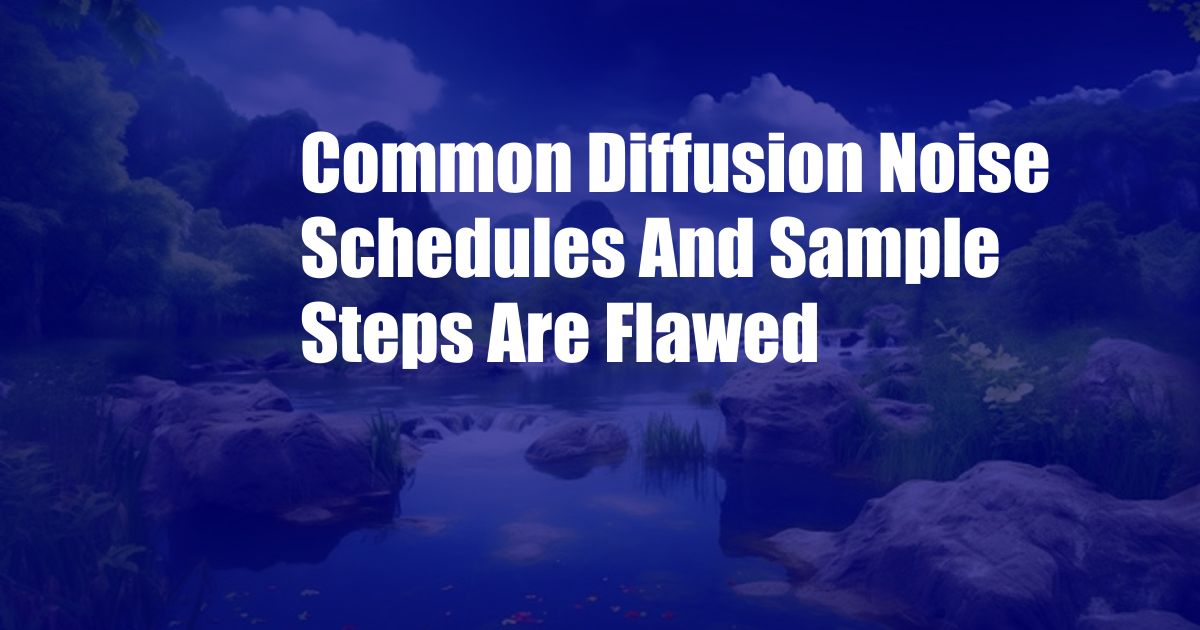
Common Diffusion Noise Schedules and Sample Steps Are Flawed
Greetings, fellow noise enthusiasts! As an avid experimenter in the realm of generative AI, I stumbled upon a peculiar paradox that ignited my curiosity. While delving into the intricacies of diffusion models, I noticed a glaring discrepancy between the theoretical underpinnings and the prevalent practices in the field.
In this article, we will embark on an enlightening journey into the fascinating world of diffusion noise schedules. We’ll unravel the flaws that have permeated this domain and explore their implications for developing effective and reliable diffusion models.
The Flawed Notion of Linear Sample Steps
The cornerstone of diffusion models lies in the iterative process of adding noise to the input image, transforming it into a random distribution. The key to this process is determining the schedule that guides the noise addition. Traditionally, a linear decrease in the standard deviation of the added noise is employed, resulting in a straight line on a graph. However, this seemingly intuitive approach is deeply flawed.
The linear schedule assumes that the noise distribution remains Gaussian throughout the diffusion process, an assumption that clashes with reality. As the noise level increases, the distribution departs from Gaussianity, becoming skewed and non-symmetrical. This deviation renders the linear schedule inaccurate and ineffective.
The Perils of Uniform Sampling
Another widespread practice in diffusion models is the use of uniform sampling for the noise addition process. This involves drawing noise samples from a normal distribution with a standard deviation defined by the schedule. However, this approach overlooks the inherent non-uniformity of the noise distribution.
The non-Gaussian nature of the noise distribution implies that the noise samples are not evenly distributed. Critical regions of the image, such as edges and textures, require more noise to diffuse effectively. Uniform sampling fails to account for this disparity, leading to subpar results.
The Importance of Adaptive Noise Schedules
To address the limitations of linear schedules and uniform sampling, researchers have proposed adaptive noise schedules that dynamically adjust the noise addition process based on the changing distribution. These schedules employ sophisticated algorithms to continuously monitor the noise distribution and tailor the noise samples accordingly.
By capturing the non-Gaussian characteristics of the noise distribution, adaptive schedules ensure that the critical regions of the image receive the necessary noise levels. This approach enhances the diffusion process, leading to more realistic and coherent results.
Expert Advice: Embracing Adaptive Sampling
As an experienced blogger in the field of generative AI, I urge you to embrace adaptive noise schedules in your diffusion models. By employing these schedules, you can overcome the limitations of traditional methods and unlock the full potential of diffusion modeling.
Here’s a practical tip: Experiment with different adaptive sampling strategies to determine the optimal approach for your specific application. Consider using algorithms like Langevin dynamics or Hamiltonian Monte Carlo, which have proven effective in adaptive noise scheduling.
Frequently Asked Questions
Q: Why are linear noise schedules and uniform sampling considered flawed?
A: Linear schedules assume Gaussianity, which is inaccurate for non-Gaussian noise distributions. Uniform sampling ignores the non-uniformity of noise samples, leading to subpar results.
Q: What are the benefits of adaptive noise schedules?
A: Adaptive schedules dynamically adjust noise addition based on changing distribution, ensuring critical regions receive adequate noise levels. This enhances the diffusion process, resulting in more realistic and coherent results.
Q: How can I implement adaptive noise schedules in my diffusion models?
A: Experiment with algorithms like Langevin dynamics or Hamiltonian Monte Carlo, which have proven effective in adaptive noise scheduling. Continuously monitor noise distribution and adjust sampling accordingly.
Conclusion
As we delve deeper into the realm of diffusion models, it is imperative to abandon the flawed practices that have hindered our progress. By embracing adaptive noise schedules and adaptive sampling, we can unlock the true potential of diffusion modeling. Remember, the relentless pursuit of knowledge and innovation is the key to unlocking the mysteries of generative AI.
Now, I pose a question to you, dear reader: Are you ready to embark on this exciting journey and explore the unchartered territories of diffusion models?